HOW TO (start a) SEARCH FOR TRUTH
Eric
Lormand
University
of Michigan
lormand@umich.edu
Draft:
December, 2001
I. AIMING FOR TRUTH
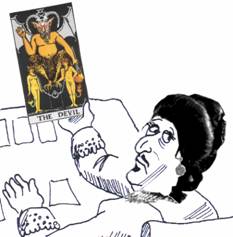
|
Meet longtime Tarot reader and renowned occultist Renée
O’Cards. Wracked with guilt over her epistemic irresponsibility, seized with
fear of being deceived by a malignant demon, and prone to escape into sleep
and dreams for unknown time periods, she turns to the consolation of First
Philosophy. Today, in secure possession of leisure in a peaceable
retirement, she opportunely frees her mind from all other cares, is happily
disturbed by no passions, and at length applies herself earnestly and freely
to Seeking Truth.
Unfortunately,
the First Philosophers she meets advise her to accept whatever Method, at
root, is easiest or makes her generally happiest.
She discards such a merely “pragmatic” search, offering her crass
advisors the use
of her old client list, since Tarot readers these days get easy money and a
loving network of Psychic Friends. Her search is to be purely “epistemic”, based singlemindedly
on her aim for Truth.
How
should Madame O’Cards … well, she’s discarded her professional name … how
should Renée proceed?
|
Of course when Renée aims for
“truth” she is not on a hunt for the abstract property of being true, itself.
She aims for true things. And she aims “for” true things in the sense of
aiming to be poised (or inclined) to use them as true, to take
advantage of their truth—for instance, to have true beliefs.
She can use true beliefs in order to reach more true beliefs. Or in case she
ever comes to be disturbed by a passion, potentially she can use true beliefs
in figuring out how to indulge it. But this latter merely practical use does
not constrain her purely epistemic aims.
Renée might form true beliefs too
easily to satisfy herself, by “covering all the bases”, that is, accepting each
candidate belief along with any apparently competing candidates (perhaps she is
poised to use each of them as true, but in different circumstances or for
different purposes). This would be unsatisfying because she also seeks nothing
but the truth—so that there is a truth that p for each of her
beliefs that p—and her aimings allow for the alleged possibility of
untrue beliefs.
Similarly, Renée might form some
true beliefs too easily to satisfy herself. She might leave out a lot of
truths, and there are no true beliefs she isn’t aiming to form. Ideally she
seeks the whole truth—a “complete” group of true beliefs, a belief that
p for every truth that p.
I’ll say Renée’s target is “Truth”
(capital “T”) as a shorthand in two ways for what she really aims for—a
shorthand for true beliefs, and a shorthand for the truth, the whole
truth, and nothing but the truth.
On the
other hand, her overall aiming for Truth is not all-or-nothing: it is more
satisfied the more true beliefs she has and the fewer untrue beliefs she has.
Her basic
overall Truth-aim factors into two kinds of aims, each with conditional
content. She aims that for each candidate belief that p: if p,
then she believes p; and if she believes p, then p. (See the central root of
the tree in the Appendix, Aim 1.)
II. AIMING FOR EPISTEMIC
RESPONSIBILITY
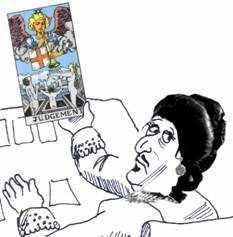
|
Renée’s
fear of a falsity-insuring malignant demon makes her fantasize about a
truth-insuring benign angel. But perhaps surprisingly, that also scares her!
She aims to find truth, proudly, versus being given it, luckily.
She aims to be responsible for reaching Truth: to develop a set of
sub-aims that she can satisfy, and that lead to satisfaction of her ultimate aim
for Truth.
The
problem is that any old aim A leads to Truth if an angel rewards those who
aim for A—e.g., by revealing Truth to them on Judgement Day. And any old aim
A precludes Truth if a demon punishes those who aim for A—e.g. by massively
deceiving them. So even if outcome O is necessary for Truth, aiming
for O seems to require betting against demonic punishment for O-aimers. And
even if outcome N necessarily precludes Truth, aiming against N seems to require betting against angelic rewards
for N-aimers. But Renée has no evidence against such demons and angels.
Deep inside Renée, however, is
a Nordic defiance of the Fates. She does aim for Truth. But if demons punish
her otherwise rational sub-aims, she’d prefer to sacrifice Truth, and to
reason as if she can satisfy these sub-aims. Nor would she prefer to sell out
her reason to angels promising Truth. How heroic!
|
Even
if conditions don’t allow her to be causally responsible for reaching
Truth, Renée resolves to reason responsibly. This is a kind of virtual
or representational responsibility: to specify sub‑aims that serve her
end-aims if conditions don’t block her from satisfying the sub-aims.
Specifically epistemic responsibility is reasoning responsibly in this
sense, and doing so based on the sole initial aim for Truth. (Though
Renée doesn’t take epistemic responsibility as a means to Truth, her aim
for epistemic responsibility is still “based” on her aim for Truth in a second
sense: her aim for epistemic responsibility depends on the existence of
her aim for Truth. She wouldn’t have an aim for rational responsibility unless
she had the aim for Truth.) (See the rightmost root
of the tree in the
Appendix, Aim 2a.)
Renée has a core ground rule for
prideful epistemic responsibility. It constrains her cognitive starting point.
She aims “carefully to avoid precipitancy and prejudice” where she “cannot
exclude all ground of doubt” (see Discourse on the Methods of Rightly
Conducting the Reason, and of Seeking Truth in the Seances; also Aim 2b of
the
Appendix.) I will suppose that with one
kind of exception, Renée is unwilling initially to accept contingent
candidate beliefs as (probably or actually) true or (probably or
actually) false. She will not accept such candidate beliefs without
epistemically responsible reasons, and at the start she doesn’t have such
reasons. But as I said there is one kind of exception: beliefs of the form “I
think p” about the contents of her (candidate) beliefs and aims.
Although it is contingent which beliefs and aims Renée has—just as it is
contingent whether she even exists—I will assume she correctly forms contingent
self-directed beliefs about them. Also, I will assume she does
correctly sort (candidate) beliefs as necessarily true or as necessarily
false. I will try to show how she
can proceed from there to a justified method of searching for (further)
contingent truths. My hope is that this will eventually point to a way to reach
the same end from a more meager starting point, one without initial reliance on
self‑directed beliefs or beliefs about necessities.
III. AIMING TO SEARCH
Renée
aims to search for Truth. What can she build into this aim, without prejudice
about what’s contingently true? She can stick to necessary parts of
searching in general, independent of the specific Truth-search:
i.e., general facts about searching for food, shelter, justice, peace, love,
hate, war, … even falsity. Then she can remain unprejudiced by applying only
those general necessary parts to her Truth search.
In addition to an aiming for X,
conducting a search for X requires a stock of possible candidates for X,
and a kind of accepting of candidates. Accepting a candidate X can be
various things in various searches: acting as if the candidate X is an X, using
it for aimings that are satisfied only if it is an X, believing that it is an
X, transforming it into an X, etc., or some combination of these. In some
cases, acceptance of a candidate for X varies in degree, along one or more
dimensions. For example, you may be more or less inclined to trust something
you accept as a healthy food, even holding fixed your aiming to eat and your
opportunity. Or you may be much inclined to use an accepted shelter to avoid
snow but not to avoid bombs. Degrees of acceptance may be precise or rough,
quantitative or qualitative.
A candidate that is not accepted to
any degree is rejected. In some cases the target of the search and the
acceptance mechanisms of the searcher place no effective limits on how many
candidates can be accepted. But in other cases candidates compete for
acceptance, because of total limits on the number of acceptances or because the
aimings or capacities of the searcher set up specific pairwise tensions among
candidates. For instance, you might set up a competition among your candidate
charitable recipients, but you’d be less likely to set up a competition among
your candidate charitable donors.
Candidates are accepted (or
rejected) according to a test for X,
whose reliability may fall anywhere from infallible to routinely misguiding or
even necessarily misguiding. If there are distinct mechanisms for testing and
for accepting, the immediate results of the test are scores—aimings to
accept or reject—that typically in turn cause acceptance outcomes unless
something interferes with the acceptance mechanisms. (If somehow there aren’t
distinct mechanisms for testing and for acceptance; then the test scores are
the acceptance outcomes rather than mere aimings for or causes of
acceptance.) Scores have a number of dimensions and a degree of precision to
match the search’s various possible dimensions and degrees of acceptance.
Without loss of generality, I’ll assume that the higher the score (perhaps above some threshold), the more the
candidate is accepted.
The process by which possible
candidates become subject to testing is generation
of candidates, which may be blind (e.g., randomly or unthinkingly stumbling
upon a candidate) or guided (e.g., creating a candidate by design). Unlike
passing or failing a test, which may admit of degrees, generation of candidates
is all‑or‑none: a candidate is either tested or it isn’t. In some
cases, a test relies on an exhaustive group of possible candidates’
being available simultaneously. The test is applied once to the whole group,
and the search halts. In other cases, a test is able iteratively to test
possible candidates as they become available, nonexhaustively, perhaps even one
candidate at a time. Iteration matters if the test can be modified by provisionally
accepted candidates, candidates that are accepted to some degree but are
subject to further testing and potential rejection (e.g., if a competing
candidate scores higher in subsequent loops through the search steps). In that
case, the order in which candidates become available may affect the outcomes on
subsequent iterations, by affecting which candidates are provisionally accepted
while the search continues. The search is halted
when a candidate gets a special (e.g., perfect) test score, or when there are
no new possible candidates, candidates
that have not already undergone the same test.
So in the general case,
a search for X contains an ordered series of four steps (which may be
repeated):
(GX) Generating new
candidates for X.
(TX) Scoring generated
candidates according to a test for being X.
(AX) Accepting a
candidate to the degree it scores highly on the test (absolutely or relative to
competing
candidates).
(HX) Halting if a
candidate scores highly enough on the test or if there are no new candidates,
otherwise
looping
to (GX).
A method of
searching for X is a particular means for performing (GX), (TX),
(AX),
and (HX)—including
a generation procedure, a test, a kind of accepting, and threshold scores for
accepting and for halting. (See the leftmost root of the
Appendix tree, Aim
3a.)
Since Renée’s aim for Truth is
partially satisfiable by less than the whole truth, she doesn’t aim merely to
mount a single search for the whole truth, rejecting candidate groups of
beliefs simply because they are incomplete. Instead, she aims gradually to
aggregate subsearches, each aimed at finding some (possibly
incomplete) truth(s). She aims to subsearch and aggregate until, ideally, her
aggregate is complete. (In Renée’s terms she aims “to divide the problem of
seeking truth into as many parts as possible” and “to ascend step by step from
the easiest parts to the most difficult parts” and “to enumerate these steps so
completely … that nothing is omitted”.) (See Aim 3b of the
Appendix.)
Applying
only the necessary parts of searching in general to her search for Truth, Renée
aims to mount (sub)searches for truth with the following steps:
(GT)
Generate new candidate beliefs.
(TT) Score generated candidates according to a
test for being true.
(AT)
Accept a candidate to the degree it scores ….
(HT)
Halt …, or else loop to (GT).
In the specific context of (AT),
accepting a candidate belief that p is forming a belief that p.
If the threshold score for halting is higher than that in the previous step for
accepting, Renée may form a belief provisionally without halting the search,
and might reject it—destroy it—if another candidate belief scores higher in
subsequent iterations of the search steps. Renée’s
test at (TT) may depend on candidate beliefs she accepted
provisionally at a prior (AT) in the same subsearch, or on her
aggregated beliefs from prior subsearches.
IV. AIMING TO METASEARCH
Though
Renée aims to search for Truth, she aims first to search for a method of
searching for Truth. And not just any old method, of course; her aim, ideally,
is to find an ideal method of searching for truth, where what’s ideal is
measured by her aim of finding Truth. Or, failing that, it’s best if she finds
the best method of searching for truth, the method that is closest
to the ideal, where closeness is also measured by her aim of finding Truth. Or,
at least, it would be alright if she finds a right method of searching
for truth, a method that is better than some other candidate methods she will
have considered, and no worse than any others. She aims to mount a “metasearch”
for Truth, which is a search for a right (/best/ideal) method of searching for
Truth.
As
before, to avoid prejudice she should carry over to the metasearch for Truth
only necessary parts of metasearching in general. A metasearch for X
follows steps similar to a search for X, but with “a right method of searching
for X” substituted for “X”:
(GM)
Generate new candidates for a right method of searching for X.
(TM)
Score generated candidates according to a test for being a right method of
searching for X.
(AM)
Accept a candidate to the degree it scores ….
(HM) Halt …, or else loop
to (GM).
In this case
“accepting” a candidate method is being poised to use the method in searching
for X.
Putting aside
issues of feasibility, an ideal method of searching for X—ideal for the aim of
finding X, setting aside any other ideals—would meet three specifications, or
what I’ll call “X-Specs”:
X-Coverage:
X is among the candidates generated and tested by the search.
X-Discrimination: X scores
perfectly, or better than all possible competing candidates, and so is
accepted.
X-Stability:
X remains accepted because its score halts the search or remains higher than
any subsequent
competitor’s score.
What’s more, an
ideal search method—with heavy emphasis on “ideal”—would meet these X-Specs necessarily,
no matter how the world is. Such a search method would in every possible
circumstance enable one to find X.
In case no ideal candidate method is
feasible, less than ideal methods might at least partially satisfy the root aim
of finding X. Other aims aside, one feasible search method for X is better
than another feasible search method for X the closer it approximates to the
ideal. There are several interesting dimensions of proximity to the ideal case,
based on the degree to which they satisfy the aim of “finding” X. One dimension
varies the necessity of a perfect method’s meeting the X‑Specs.
One method might be better than another in meeting the X-Specs with greater probability
given specific actual conditions. Another dimension of proximity to the
ideal varies a perfect method’s jointly meeting the three X‑Specs.
In some possible condition, imperfect methods might meet only X-Coverage and X‑Discrimination
without X‑Stability, or might meet only X-Coverage without
X-Discriminating and X-Stability, or might meet none of these.
The more X‑Specs a method meets in circumstance C, the better the method
is at finding X in C.
So if C is the actual or probable conditions surrounding the search, the more
X-Specs a candidate method meets in C, and the more probably it meets them in
C, the higher its score in the metasearch.
The crucial problem is how to test
whether it is the aimed‑for target X that a candidate method
covers, discriminates, or stabilizes on in conditions C. One way to do this is
to compare the candidate method’s scores with unquestioned scores already given
to candidates for X. For example, if a candidate food has already been scored
(accepted to some degree or rejected), it can be used to “calibrate” candidate
methods in the metasearch for a right method of searching for food. A method’s
own score is higher the closer the match between (a) the food’s actual score
and (b) the score a candidate method gives to that candidate food. A second way
uses beliefs about general features of food, even if no specific candidate food
has already been accepted or rejected. The higher the score a candidate method
gives to candidates with those general features, the higher the method’s own score
in the metasearch.
Call
the process of calibrating one’s method using already accepted candidates for
X, or using substantive beliefs about general features of X, the “Circle of
Calibration”. Can one metasearch for X from scratch—without going around
this Circle? It seems that one cannot. Usually this circularity is
little or no hindrance, because we do already have accepted candidates
for X or substantive beliefs about X before we metasearch—usually it’s these
morsels that give us the ideas or aims about X in the first place, and usually
they go unquestioned.
Now let’s apply
this in the context of Renée’s search for Truth. A metasearch for Truth
contains the following four (repeatable) steps:
(GR)
Generate new candidates for a right method of (sub)searching for Truth.
(TR)
Score generated candidates according to a test for being a right method of
(sub)searching for Truth.
(AR)
Accept a candidate to the degree it scores ….
(HR)
Halt …, or else loop to (GR).
Judged solely by her
aim of finding Truth, her ideal method of searching for Truth would satisfy the
following three “Truth‑Specs” (special cases of the “X-Specs” listed
above), and would do so necessarily:
Truth-Coverage: If p,
then a candidate belief that p is generated and tested.
Truth-Discrimination: If
p, then a candidate belief that p scores perfectly or better than
competitors,
and so is
accepted.
Truth-Stability: If p, then a
candidate belief that p remains accepted because its score halts the
(sub)search
or remains higher than any subsequent
competitor’s score.
(See the trunk of the
Appendix
tree, Aims 4-6.) Before factoring in the points about less-than-ideal cases and
circular calibration, let’s look at Renée’s bold push for the ideal …
V. CARDESIAN FOUNDATIONS
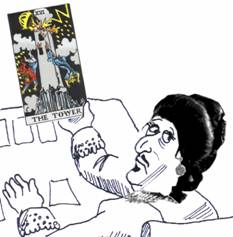
|
Obsessed by her
newfound devotion to philosophical rigor, Renée frees up the rest of the
week—not one day less!—for finding an ideal truth‑searching method. On
the second day she reasons that since she’s thinking, she exists. But this
does little to quench her thirst for the whole truth. On the third and fourth
day she argues for the existence of a perfect God (who necessarily protects
her from massive deception)—basically, if there weren’t such a perfect being,
she wouldn’t even have an idea of a perfect being, since she couldn’t have
gotten the idea of perfection from thinking about imperfect things. Over the
next couple days she tries to build a tower toward the truth, the whole
truth, and nothing but the truth, “so help me God”. (For details of this deal
with the divinity, see Meditations on Faust Philosophy.)
Evening of the sixth day, she sees all that she had
made, and, behold, it wasn’t very good. Even given the assumption that she
exists and thinks, on second thought she realizes her idea of a perfect being
could’ve come from combining ideas of imperfection and of negation, each
honed from thinking about imperfect beings. And anyway, she realizes that
even a perfect God might deceive her—maybe being massively deceived builds
character. Nearly a whole week’s edifice of meditations committed to the
flames.
|
Ah, well. In the absence of an ideal
method of searching for Truth, Renée’s now ready to settle for a method that
merely approximates the ideal. Comparisons of methods as better or worse can be
derived from the general features of X‑Specs. As in the general case,
judged by the aim of finding Truth, in circumstance C one method of searching
for Truth is better than another the more Truth‑Specs it satisfies in C,
and the more probably it satisfies the Truth‑Specs in C. But how can
Renée test whether it is truth that a method covers, discriminates, or
stabilizes on in a given context? Her search is for contingent truths beyond
those of the self-directed “I think p” variety. But initially Renée
makes no use of accepted candidates for such truths or substantive (contingent)
beliefs about the general features of such truths. This aversion to prejudice seems to prevent
her from calibrating methods according to whether they cover, discriminate or
stabilize on such truths. So it seems she can’t use the Truth-Specs to assess
methods of forming such true beliefs, initially. Perhaps the Truth-Specs will
be of use if her subsearches ever get rolling, and she reaches some more useful
contingent beliefs. But initially the Circle of Truth‑Calibration
is unbroken.
But remember that “truth” in Renée’s
aims is shorthand for true beliefs. One potential workaround for
Renée—and the only one that occurs to me—is to pare away from the Truth-Specs
issues about whether the relevant beliefs are true, leaving pared-down Specs
about beliefs independent of their truth or falsity. For example, unless
there are some candidate beliefs (whether true or false) that a method
stabilizes on, it doesn’t stabilize on true beliefs. Unless there are some
pairs of competing candidate beliefs (whether true or false) that a method
discriminates between (i.e., accepting one and rejecting the other), then it
doesn’t discriminate true beliefs from false beliefs. And unless
there are some candidate beliefs (whether true or false) that a method
covers, then it doesn’t cover true beliefs. Furthermore, if a method is,
ideally, to cover truth in all possible conditions, ideally it should be
capable of covering any possible beliefs (this also seems to be required
for avoiding prejudice). So part of Renée’s aims for Truth-Coverage, Truth‑Discrimination,
and Truth-Stability are aims for the following Belief‑Specs:
Belief-Coverage:
Each possible contingent candidate belief is potentially generated and tested.
Belief-Discrimination:
Some candidate beliefs score perfectly or best among their competitors,
while
other competitors do not score as high, and so the former (and not the latter)
are accepted.
Belief-Stability: Some candidate
beliefs remain accepted, because their scores halt the (sub)search, or remain
higher than
any subsequent competitors’ scores.
(See the branches of the
Appendix
tree, Aims 7-9.) Does Renée have trouble breaking the Circle of Calibration as it
applies to beliefs? She does start out with examples of contingent candidate
beliefs—it doesn’t matter here that she doesn’t start out assuming which of
them are true, and that she doesn’t start out with them as contingent accepted
beliefs. And perhaps she starts out with beliefs about some general features of
contingent candidate beliefs—assuming there are features necessary to
contingent candidate beliefs, which she keys on to distinguish contingent from
noncontingent candidates in the first place. Either way, she may be able to
calibrate candidate methods according to whether they meet the Belief-Specs.
And when beliefs that are covered, discriminated, or stabilized on
happen to be true—even if this is unbeknownst to Renée—satisfying these
Belief-Specs satisfies the Truth‑Specs. By carefully clearing out the
issues specifically about Truth, we reach ground upon which Renée may be able
to build without prejudice. But there is a little more ground‑clearing to
do.
As
in the general case, there are a couple legitimate dimensions along which a method
can approximate the ideal of necessarily meeting the Belief-Specs. Even
short of the ideal, one method is better than another if it actually
meets the Belief-Specs while the other merely possibly does. This
standard of actuality may be important to Renée eventually, but since she does
not start out with prejudiced assumptions about what’s actual versus merely
possible, she cannot use the standard initially to discriminate among
methods. (She
might just wait around until some possible Judgement Day to compare
retrospectively how candidate methods “did” in the actual world. But that would
be pointless given her aim of finding truth.) Leaving aside
whether the methods actually meet the Belief-Specs, one method is better than
another if it more probably does so. This standard of probability may
also be of eventual use to Renée, but it is of no initial use: she makes no
prejudiced assumptions about what’s probable. So initially Renée seems limited
to comparing methods according to whether they do or don’t necessarily
meet the Belief-Specs.
Trouble is, as
Renée realizes, no method necessarily meets the Belief-Specs, since for
each method a possible demon could be bent on punishing users of that method.
She also realizes that no method necessarily fails to meet the Belief‑Specs,
given possible angels bent on rewarding use of any given method. But here’s a
place where Renée’s aim for epistemic responsibility kicks in. She can accept
sub-aims for her Belief-Spec-aims in this way: by reasoning about what would necessarily
lead to the Belief-Specs if she is able to satisfy the candidate
sub-aims. This, finally, requires no more than her permitted cognitive starting
point of beliefs about necessities (and possibilities) and self-directed
beliefs about her beliefs (and aims). But can she use it to justify aims for
one method rather than another?
VI. AIMING FOR COVERAGE
Renée’s aim for
Belief-Coverage is an aim that each possible contingent candidate belief is
potentially generated and tested. Here is how she can use this aim to
assess truth-searching methods with respect to their procedures for generating
candidate beliefs.
TOLERATING NONEXCLUSIVE GENERATION (Aim 10)
For purposes of filtering all
possible candidate beliefs down to the true ones, it might seem that the
distinction between generating and testing is arbitrary—being excluded from
testing in a generation stage seems equivalent to being included as a candidate
but failing in a testing stage. Even a search with the most lenient or
inclusive kind of testing phase (“accept all candidate beliefs”) successfully
finds truth if a strict or exclusive enough generation phase compensates—if
only true candidates are generated. Even a search with the most lenient or
inclusive kind of generation phase (“test all possible candidate beliefs”)
successfully finds truth if a strict or exclusive enough testing phase
compensates—if only true candidates pass the test.
No matter how the filtering is done,
Renée’s aims for epistemic responsibility are satisfied only if she takes
responsibility for each bit of filtering. Ideally, she divides the filtering
task into the smallest possible filtering processes, orders them from coarsest
to finest, keeps track of them all, and in her metasearch evaluates each. Any
unevaluated filtering process is
prejudiced, and if it nevertheless succeeds in filtering out false candidates,
this is a matter of epistemic luck which Renée proudly aims to avoid.
Without loss of generality, any
filtering process can be treated as part of her test for truth rather than part
of her initial generation phase, as can any process of evaluating candidate
filters. Collapsing these into her test for truth, the only way she can avoid
prejudice is to have a test that can work even given a maximally inclusive or lenient generation
phase, because if she excludes possible candidates from generation, without
even giving them a “hearing” and without even having evaluated such exclusions,
her method is prejudiced. So she aims to find a test that is not a mere
rubber‑stamp, but which leads to truth even given a non-exclusive
generation phases. Her ideal test “tolerates” non‑exclusive generation.
Her purely epistemic search isn’t disturbed by passions for saving time and
effort, so she has no initial countervailing reason to opt for an exclusive
generation phase.
TOLERATING NONEXHAUSTIVE
GENERATION
(Aims 11-12)
It might
seem that an ideal generation procedure for Renée, given that she is not in a
hurry, is to generate every possible candidate belief, and then test them all
together as a batch. That would be an ideal generation phase, but equally, an
ideal test would tolerate a less-than-ideal generation phase. If Renée
generates a candidate that she does not bother testing, that would be a kind of
prejudice. So Renée aims ideally for testing each candidate she
generates. If her test requires a batch of candidates to be generated
before it can be applied to any of them, she might unluckily halt (e.g., die,
or otherwise get bogged down endlessly) in the middle of a generation phase. So
the further her test is from requiring exhaustive generation, the less
she relies on luck and the more she takes responsibility for testing each
generated candidate. She aims ideally for a test that can work at each
iteration with small batches of competing candidates, even applying to them
one-by-one, immediately upon generation. Only if she has such a test, and uses
it that way, can she necessarily test each generated candidate. So her ideal
test would tolerate one‑by‑one generation phases.
TOLERATING RANDOM GENERATION (Aim 13)
Now
if a nonexhaustive set of candidates is to be tested without prejudiced
exclusivity, then an ideal test for Renée would work even if candidates are
generated randomly, at least from among the possible candidates not
already put to the same test. So,
epistemically responsibly, she aims ideally for a test that can tolerate random
generation. (This aim could be satisfied even if Renée actually has an
exclusive or exhaustive or nonrandom generation phase; she can be proudly
responsible so long as her test does not rely at the start on such
generation.)
VII. AIMING FOR DISCRIMINATION
Renée’s aim for
Belief-Discrimination is an aim that some candidate beliefs score perfectly or
best among their competitors, while other competitors do not score as high. Here
is how she can use this aim to constrain the general features her scoring
factors should have. When she is done, she will be in position to start her
metasearch.
NONNEUTRAL SCORING FACTORS (Aim 14)
Renée
aims ideally to have some factors or other that raise or lower scores;
otherwise, all beliefs get the same scores. No nonneutral factors, no
(responsible) discrimination among competing beliefs.
ROBUST SCORING FACTORS (Aim 15)
It’s
possible for there to be truths that are independent of the (perhaps random)
order in which Renée generates candidates. Since she aims to discriminate even
such truths, she aims ideally for a test that can give the same scores to the
same candidates in the same conditions, no matter the generation order.
Ideally, acceptable candidate beliefs are robustly acceptable, across
variations in generation.
SEMANTICALLY RELEVANT SCORING
FACTORS (Aim
16)
Let
C be a (contingent) true candidate belief that p. Suppose Renée were to
score C only by using features that are completely independent of whether C is
true. Also suppose that the independence holds in both directions—that C’s
truth or falsity is completely independent of the features Renée uses. Despite
the mutual independence, such features could be contingently correlated
with C’s truth or falsity. But at the start of her search, Renée has no beliefs
in such contingent correlations. At best she is epistemically lucky if she
discriminates whether C is true, using only such features. So at least
initially she aims to score C using features that are in a dependence
relation with C’s being true or with C’s being false.
Now
suppose that Renée were to score C only by using features that are in a merely contingent
dependence relation with C’s truth or falsity. Then similarly, at least initially
when she lacks beliefs in such contingent connections, she could at best be
lucky if she discriminates whether C is true. So at least initially, she aims
ideally to score C using features that are in a necessary dependence
relation with C’s truth status.
What
sorts of features could she use, initially? Whether C is true depends
necessarily on (i) C’s having the content that p, and on (ii) whether p.
Given her contingent self-directed beliefs about the content of her (candidate)
beliefs, she may be able to use (i), and other contingencies to which (i)
stands in a relation of necessary dependence.
But beyond this, feature (ii) is no help: prior to accepting C, Renée cannot
make use of (ii), and at the start of her search, she cannot make use of any
other contingency even if it is in a necessary dependence relation with (ii).
So Renée aims to score C, at least initially, using features on which C’s
content necessarily depends or which necessarily depend on C’s content. In
general, initially she aims ideally to use scoring factors that are necessarily
semantically relevant. This aim steers her away from initial reliance on
intrinsic “hardware” features of beliefs (physical parts, size, etc.), and away
from extrinsic physical or causal features of belief (physical location,
contingent or context‑dependent use, etc.). These don’t have the kind of
necessary connection to content that might enable epistemically responsible
discrimination.
NONRANDOM SCORING FACTORS (Aim 17)
First, consider
a tempting but unsuccessful argument against random scoring: it seems that
random scoring either prevents discrimination (when scores for all competitors
happen to match) or else makes Renée merely epistemically lucky to discriminate
(when scores for all competitors happen not to match). The loophole in this
argument is that discrimination can be assured, responsibly, by
assigning scores randomly except for scores that are already assigned.
(This is akin to drawing lots randomly “without replacement”—one draws a
different lot each time.) A better way to motivate an aim for nonrandom scoring
is via the aim for semantically relevant scoring: genuinely random scoring
depends on no specific features at all of the beliefs scored, and so depends on
no features that are semantically relevant to the specific beliefs scored.
VIII.
A METHOD FOR RENÉE’S METASEARCH
Renée aims to
find nonneutral scoring factors, ones that raise or lower scores of candidate
beliefs. Since the main point of finding factors is to score contingent
candidates, and since she doesn’t start out with any accepted contingent
beliefs (beyond the “I think p” sort):
Ideally,
the right factors should adjust the scores of contingent candidates
even in the absence of previously
accepted contingent candidates.
She can also apply her structural aims for ideal generation and
scoring as follows:
Ideally,
the right scoring factors
should outscore competitors robustly,
independently of order of
generation. (from Aim 15)
Ideally,
the right
scoring factors should outscore a competitor even if tested one versus one.
(from Aim 12)
Also, since Renée doesn’t start out with any accepted scoring
factors, she aims to make a first acceptance (perhaps provisionally), so
Ideally,
the right scoring factors should outscore competitors
even in the absence of previously accepted
scoring factors.
These general features provide ways to score candidate scoring
factors.
To check for
these features Renée aims to reason about a variety of possible testing
contexts (with different orders of generation, different batches of
competitors, and different previously accepted beliefs). What are her
resources? Given the aim for epistemic responsibility, she only needs to reason
about how the tests would proceed in these contexts if their component
individual aims are satisfied. (So no fair meddling demons, meddling angels,
and unluckily falling anvils.) And without prejudice, she can use beliefs about
what is or isn’t necessarily true or false if the tests proceed as
aimed. Here’s how she could responsibly proceed.
Step One
(Generating): Think of some candidate factors—it doesn’t matter how Renée does
this … randomly, or exhaustively, or by focusing on necessarily semantically
relevant dimensions characterizing all or many candidates.
Step Two (Minimal
Testing): For each candidate factor F, consider a minimal testing
context in which F is the only generated factor, tested alone.
There are three “basic” candidate aims about how candidate factor F applies
(overall, or at first):
FVirtue
= F raises scores;
FVice
= F lowers scores;
FNeutral
= F doesn’t affect scores.
Score these aims by whether under the minimal conditions they necessarily
insure or preclude responsible coverage, discrimination, or stability (of
belief). FNeutral’s score is lowered because in the absence of other
factors, it (necessarily) precludes (responsible) discrimination. Accepting FNeutral
in this context would leave Renée without any way to pull apart the scores of competing
beliefs. So if either FVirtue or FVice outscores the
other, it wins this three-way competition, so F is provisionally acceptable as
a scoring factor in the minimal testing context.
Step Three
(Robust Testing): For each provisionally acceptable factor F from Step Two,
consider whether F would be robustly acceptable in more elaborate testing
contexts, especially when other surviving factors have been previously accepted
(so the aim of having some scoring factor does not automatically lower FNeutral’s
score), when F itself has been previously accepted, or when F is considered not
alone but in competition or in conjunction with other factors. Strengthen or
weaken acceptance of F to the degree it is or isn’t robustly acceptable.
Step Four (Contingent
Testing and Iterating): Start the search for (more of the) Truth (i.e., the
aggregation of subsearches for incomplete truths), if at some point enough
provisional scoring factors are in place to enable some contingent candidate
beliefs to be provisionally accepted over competitors. Adjust the factors
accepted in the metasearch as may be required in light of the ongoing search
(and the provisional metasearch). This could be done by generating new
candidate scoring factors (as in Step One), then by considering nearly
minimal testing contexts (as in Step Two, except that the new candidate scoring
factors are tested one‑by-one in the context of Renée’s actual search—her
provisionally accepted scoring factors and provisionally accepted beliefs),
then by testing robustness of the scoring factors within this provisionally
growing context (as in Step Three). Renée has no need to halt her metasearch
until she halts her search; the two can run in parallel. All that’s required to
start the search are provisional, rather than final, results from the
metasearch.
IX. AIMING FOR STABILITY
Renée’s aim for
Belief-Stability is an aim that at some point a perfect or best
(discriminating) score for a candidate belief halts the (sub)search, or remains
higher than any subsequent competitor’s score. Here is how she can use
this aim to give purely epistemic (provisional) support to some more-or-less
familiar candidate virtues often considered to be merely pragmatically
supportable, at best.
SIMPLICITY (Aim 18)
Step One (Generating): Renée would not responsibly
satisfy her aim for necessarily semantically relevant scoring factors by scoring beliefs according to
abundance of spatiotemporal parts or other intrinsic physical features. But she
might responsibly satisfy this aim by scoring beliefs according to abundance of
some kind of semantic “parts” or determinants. What sort of determinants could a belief have that
necessarily affect its content (in a way that potentially affects its truth or
falsity)? She might get the idea of scoring a belief in terms of its amount of
involved concepts, conjuncts, or disjuncts. Beliefs do
have different contents if determinants such as these have different contents.
However, one problem is that amount of conjuncts and disjuncts can be varied
without varying content: e.g., Renée’s (candidate) belief that p is
equivalent to her belief that p and (p or q), or her
belief that p or (p and r) or (p and s). Perhaps this can be avoided by
counting only mutually
independent conjuncts and mutually independent disjuncts. Another problem is
that amount of involved concepts can be varied without varying content: Renée’s
belief that o is a square may be equivalent to her belief that o is
an equilateral rectangle, but the former may involve more concepts than the
latter (if her concept square involves these other two concepts but not
vice versa). Perhaps this can be avoided by counting only her most basic
concepts; ones that do not involve other concepts, as her concepts are
organized.
For brevity, let’s lump all these considerations under the label “simplicity”—a
candidate belief (or group of beliefs) is simpler the fewer basic concepts it
involves and the fewer mutually independent conjuncts/disjuncts it involves.
Step Two (Minimal
Testing): In the
minimal testing context, a basic aim of treating complexity as a virtue (or
in other words, treating simplicity as a vice) would preclude responsible
stabilizing. Suppose Renée were to raise the scores of more complex
competitors. Then her (sub)search would never responsibly stabilize, since for any
candidate there is always a more complex candidate, a candidate involving more
(uses of) basic concepts or more conjuncts or more disjuncts. If it does
stabilize, it does so merely “luckily”, e.g., if Renée is hit by an anvil, or
if her generation phase illicitly excludes the more complex candidates in a
prejudiced way.
So ComplexityVice
(or in other words, SimplicityVirtue) wins, provisionally. (Recall that a scoring factor F is
ideally robust enough to be supportable in a minimal testing context, and FNeutral can only be competitive in a
minimal testing context if neither FVice nor FVirtue outscores the other.),
PARSIMONY and ANALOGY (Aims 19-20)
Step
One
(Generating): By definition, a (candidate)
belief that p is “committed” to particular contingent entities or kinds
of entities E such that, necessarily, E exists if p. Renée might
responsibly satisfy her aim for semantic relevance by considering amount of
such commitments as scoring factors. Also, a belief (or group
of beliefs) has greater “parsimony” the fewer particular entities it is
committed to.
And the fewer kinds of entities a belief (group) is committed to, the
greater “analogy” it exemplifies—the more similar to one another are the
particular entities it is committed to.
Step
Two
(Minimal Testing): Parsimony is provisionally
acceptable as a virtue for reasons similar to simplicity. Raising the scores of less parsimonious
competitors precludes stabilizing (since there is always a way to “multiply
entities” further) unless Renée gets lucky. So ParsimonyVirtue wins.
In the same way, rewarding candidates for positing more “kinds” of entities,
laws, or processes (etc.) is also a never‑stabilizing method (barring
luck). So (provisionally) the more analogous to one another the posited
entities, laws, or processes, the better.
CONSERVATISM (Aim 21)
Step
One
(Generating): Given an old belief and a new
candidate belief, whether they match in content is of course necessarily fixed by their contents. So Renée might satisfy her aim
for semantically relevant factors by scoring candidates according to amount of
matching with previous beliefs. A candidate belief with the same content as a
previous belief is “conservative”. And a
group of candidate beliefs is more conservative the more contents it shares
with previous beliefs.
Step
Two
(Minimal Testing): The minimal testing conditions in Step Two cannot quite test
conservatism; for conservatism can be treated as a vice or a virtue only when
there is a previous belief. But Renée can still test conservatism in an
unprejudiced way by imagining a testing context in which she
has previously accepted an arbitrary contingent belief that p, subject
to two provisos for avoiding prejudice. She must suppose she has accepted the belief that p without prejudice (minimally, as a result of prior testing) and she must not
use any features specific to p in testing conservatism (e.g., p
should not itself entail whether conservatism is a virtue). In this nearly
minimal testing context Renee (re)considers the belief that p along with
some rival candidate belief that q (e.g., that not p). Absent any
other reasons for choosing one rival over the other, favoring nonconservative
(/nonmatching) beliefs over conservative (/matching) beliefs means never
stabilizing, but instead oscillating forever between believing that p
and believing that q, unless luckily hit by an anvil. (First the belief that
q would be the nonmatching belief, then after it is accepted the belief that
p would be the nonmatching belief, then the belief that q again,
etc.) So ConservatismVirtue wins in the nearly minimal testing context.
Step
Three (Robust Testing): Though conservatism is supportable in minimal testing
conditions, it doesn’t fare well in the context of the other provisional
scoring factors. Renée considers a context in which simplicity (alone) is
previously accepted as a virtue, and she
considers adding conservatism as a scoring factor. The three candidate aims
ConservatismVirtue, ConservatismVice, and ConservatismNeutral seem equally simple—as
do the three corresponding beliefs, one for each aim, that it should be
accepted. ConservatismVice would still preclude responsible halting,
as in Step Two, but this is no longer sufficient to support ConservatismVirtue,
because ConservatismNeutral’s score is no longer lowered (since
Renée already has a scoring factor). So Renée should weaken her acceptance of
conservatism as a virtue (but without increasing any inclination to treat
conservatism as a vice).
X. PROSPECTS FOR RENÉE’S SEARCH
I leave open which other scoring
factors, if any, Renée can provisionally accept, prior to her search for
contingent truths. These depend on her ingenuity (if any) in generating
candidate factors (subject to constraints such as semantic relevance), on the
impact (if any) of Coverage and Discrimination as well as Stability, and on the
existence of further Specs (if any) derivable from her root aims. But since
ideas for such elaborations are scarce, I will end by illustrating how Renée’s
search might proceed given what has been supported so far.
UNIFICATION AS EXPLANATION
Simplicity, conservatism, and kin
are typically cast as criteria to be used in inference to the best explanation.
But they are supportable independently of aims for explanation. Renée
can treat them as criteria to be used in inference to best beliefs,
whether or not these have “explanatory power”. If explanatory power is a virtue
at all, perhaps it is a merely contingent virtue (to be supported as Renée aggregates
contingent truths).
CANDIDATE BELIEFS AS DATA
Renée avoids prejudice by avoid
prejudging contingencies beyond self-directed “I think p” beliefs. She
can start with beliefs about necessities and possibilities, and self-directed
beliefs about these beliefs. But where p is contingent (and not itself
of the form “I think q”), Renée doesn’t initially form the belief that
p and so doesn’t initially form the belief that she believes p.
However, she may without prejudice initially form the candidate belief that
p, and so the genuine belief that she has a candidate belief that
p. Ultimately, this is her only source of “data”.
Her key hope for going beyond this
data is to find distinctive groups of such data that can be unified by
theoretical candidate beliefs. If she generates all possible candidate beliefs
at once, or generates each through a method of reasoning of which she is
already aware, then perhaps she will not be able to find such groups. But if
some of her generation processes are partial and spontaneous instead, perhaps
she will. She must hope to unify (or in a sense, explain) why she most frequently
or readily considers candidate beliefs with such contents, why
her candidate beliefs are similar and different in these or those ways.
Maybe her spontaneous patterns of candidate beliefs are products of reliable perception,
and maybe they’re products of unreliable seances or Tarot or dreams or demonic
meddling. A method that automatically accepts all spontaneous beliefs seems
prejudiced, as does a method that automatically discriminates some from others
for acceptance, but a method that automatically rejects all of them prevents
Renée from going beyond her starting point at all. May the most virtuous
hypothesis win.
EVALUATIVE TRUTH
Renée’s epistemic reasoning is
filled with evaluations, beliefs about which candidate aims and beliefs she should
accept. But these are all hypothetical evaluations, evaluations made
true in part by her basic aim for Truth. From her starting point, without
prejudice, can she reach justified categorical evaluative beliefs,
evaluative beliefs that are true no matter what aims are in place? If
Renée’s only data are her beliefs about which candidate beliefs she has, then
she has no categorical evaluative beliefs as data (even if some of her
candidate beliefs are themselves categorically evaluative).
Perhaps categorical evaluative beliefs can help explain (or at least unify) her
nonevaluative beliefs about which candidate beliefs she has, but at the moment
Renée can’t think of how they might (or how they might do so better than
nonevaluative competitors). So suppose she has no “data” relevant to justifying
categorical evaluative beliefs.
It might appear that she is stuck.
But this is one place where it matters that her provisional method is that of
general inference to the best beliefs rather than specific inference to
the best explanation. Her aim isn’t for accepting the most
virtuous available belief that might explain or unify data. It’s to accept the
most virtuous available belief, period. If no candidate evaluative
beliefs unify any data, they are all in effect tied at unifying zero
data, so this “other thing” is “equal” and the candidates can be scored using
the other factors. Of competing categorical evaluative beliefs that equally
explain nothing, she should accept the simplest (or most parsimonious or
analogous).
Immediately (but as always,
provisionally), simplicity favors “universalist” evaluative beliefs over
“particularist” ones. For example, “everyone should do no harm” trumps
“everyone should do no harm to white people, but should do harm to black
people” … and similarly trumps other corresponding principles of racism,
sexism, ageism, patriotism, religionism, speciesism, etc., as well as egoism.
It also trumps relativism of the “weak people but not strong people should do no
harm” sort. Whatever their intuitive or pragmatic repulsion or allure, these
particularist evaluative views are provisionally epistemically irrational in
the same way as beliefs in invisible leaf-blowing leprechauns; they present a
cost in simplicity (or parsimony) without presenting a gain in explanation (or
unification). Problem is, simplicity alone does not favor one universalist
principle over another. Why shouldn’t everyone do no help rather than
doing no harm?
Suppose Renée considers these three
candidate universalist evaluative beliefs:
(A)
Ideally, every aim should be satisfied.
(B)
Ideally, some aims should be satisfied and others should be frustrated.
(C) Ideally, every aim should be
frustrated.
Candidates (A) and
(C) seem simpler than candidate (B), but equally simple to one another.
However, (C) seems to harbor a paradox in a way that (A) does not. If that air
of paradox can be spun into a reason to reject (C), then (A) is supported.
The paradox is formal if, as seems
plausible, an evaluative belief that X should exist is itself an aim for
X (see note 3 for this suggestion). In this case (C) is an aim that every aim
be frustrated, which when applied to itself would require its own frustration.
An aim for X that requires its own frustration is a self-contradictory aim
against X. And when that aim is an evaluative belief that X should exist, it is
a belief entailing that X should not exist. So (C) is a self‑contradictory
belief entailing that not every aim should be frustrated. (C) cannot be true,
so (A) wins.
Once Renée reaches belief (A), it
itself becomes evaluative “data” subject to explanation (or unification). Why
is universal aim-satisfaction ideal? Suppose Renée considers these three
candidate evaluative hypotheses:
(A1)
Each aim should be satisfied (so in the aggregate, all should be).
(A2)
Some aims should be satisfied, others are neutral (so the aggregate goes above
and beyond “duty”).
(A3) Only the totality of
aims should be satisfied (so the ideal is all-or-nothing).
(A1) is simpler than
(A2) and (A3), in not drawing distinctions among aims. It also explains (A) in
a way that is most analogous to the way basic universal facts are explained in
the nonevaluative realm. The totality of mass is subject to gravity because
each bit of mass is subject to gravity, not because gravitation is partial or
all-or-nothing.
When aims conflict, which aims
should be satisfied? Traditional utilitarian theories would appeal to some
notion of aim “strength”, but Renée
doesn’t yet see any way to support this: e.g., “stronger aims are more
important” seems no more simple than “weaker aims are more important”. Instead,
she applies the conflict resolution procedure latent in (A1): if each aim
should be satisfied, then the more aims that are satisfied, the better.
Squashing a roach, or picking a grape before its time, might frustrate a few
aims of the roach or the grape. For all we know, those aims may be extremely
strong (it is life or death for the roach and the grape, and the roach is
intensely singleminded if minded at all). Psychologically more complex beings
have more aims that would be frustrated by killing them, so (A1) favors the
life of a psychologically more complex being over the life of a psychologically
simpler being, intensity or strength aside. But the interests of groups of
simpler beings could outweigh the interests of a more complex being.
So
(A1) is where Renée gets, provisionally, when she tries to think about
evaluative matters from scratch, without prejudice, and without relying on
potentially demon-induced evaluative “intuitions”. As her methodological
metasearch and nonevaluative search develop, she might be led elsewhere. Maybe
new beliefs about basic nonevaluative facts will open up new analogies that
favor other basic evaluative facts. Maybe a version of conservatism friendlier
to intuitions will turn out to be supportable. Maybe she will reach a
contingent belief that certain thinkers’ judgments are signs of truth across
the board, and maybe they will happen to counsel against (A1). Meanwhile, you
might aim to stay away from her. But I expect she’ll be preoccupied by Seeking
Truth for too long to do you any harm.